Suppose you are a high-end ice cream seller named ‘Chilly Billy’. Your business is going well, but you have one big challenge. One of the ingredients – also your USP – is full-fat raw milk, which has a short shelf life. To ensure that you always have exactly enough milk in stock, you need to predict future sales more accurately. How? Can big data analysis be of any support?
During the Tea & Talent session How Smart is your Algorithm?, Stephan Smeekes, Associate Professor in Econometrics, and Etienne Wijler, Postdoctoral Researcher in Econometrics, unravel the myths surrounding big data. To avoid privacy violations, they rely on Chilly Billy, a fictitious ice cream brand to demonstrate the potential and pitfalls of big data analytics.
It’s not the size that matters
“Common definitions of big data are along the lines of: ‘Data sets that are too large or complex to be dealt with by traditional data-processing application software’. But, “what then”, asks Stephan Smeekes, “is too large or too complex?”
“Complexity can already exist with small datasets. And although traditional software such as Excel or SPSS, is not suitable for meaningful data analysis, more advanced software like R or Python, is already easily accessible to a broader public.” In other words, “the size of the dataset is far less important than the quality of the data itself”.
Is machine learning hot?
Once we have obtained good quality data, what is the best method to analyse it: is Machine learning hot and statistics not? “Statistics typically deals with applications containing a lot of noise, which makes it hard to find meaningful relationships based on human judgement alone. Machine learning is the part of Artificial Intelligence that studies ‘computer algorithms that improve automatically through experience’. The traditional machine-learning goal is to let the machine perform faster and more efficiently than humans.” A stylised example is provided in the figure below; a machine-learning task would focus on very fast recognition of the word UMIO in the text on the left, whereas a statistics problem would study the text on the right in detail to try and discover the original word. A promising new field, in which Stephan and Etienne are conducting research brings the two concepts together: “Statistical learning – learning and updating based on new information – bridges the gap between machine learning and statistics.”
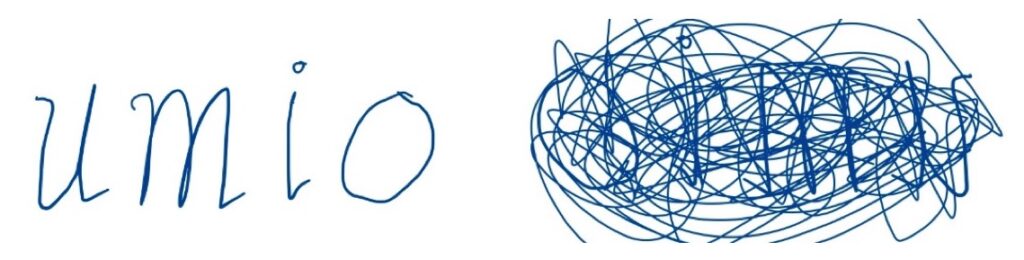
Start with data analysis in your organisation
“First of all, it is important to gather good quality data,” Stephan advises. “You also need specialised software, but not necessarily advanced mastery. The most crucial assets however, are knowledge and expertise. On the business side, this involves a profound understanding of which data are relevant to obtain the results you are after. On the analytics side, the analysts should understand the application, and be able to choose, combine and adjust existing methods to the data and application at hand.”
Smart algorithms and smart analysis
So, back to our luxury ice cream brand example, Etienne Wijler introduces the problem Chilly Billy has with the prediction of ice-cream sales. Which dataset would be sensible to look at, and which ‘smart’ algorithm should the company use? Etienne elaborates, “as for the dataset, ice cream prices and data on advertising are likely to be informative of sales. The same goes for prices and sales of the competition. Since Chilly Billy sells more expensive, gourmet ice cream, perhaps economic data offers valuable insights as well. Finally, the image and popularity of Chilly Billy is likely to play a role. Accordingly, we would recommend focussing on the collection of these variables.”
What makes an algorithm ‘smart’?
Etienne explains that a smart algorithm provides good solutions to a specific part of a problem, but that smart analysis chooses, combines and adjusts smart algorithms to solve the full problem. “Simply applying smart algorithms without thinking about the data can lead to misleading results”, Etienne says. This point is demonstrated by comparing the ice cream sales predictions of two algorithms called ‘boosting’, a popular machine-learning algorithm, and ‘SPECS’, their own algorithm. While boosting misleadingly seems to explain ice cream sales better within the dataset on which the models are estimated, it turns out to predict much worse ice cream sales on new data. Etienne explains that “while boosting is by all means a smart algorithm, it is just not smart to apply it to this kind of data that is trending over time”, highlighting the importance of smart analysis. “Smart analysis can go beyond the prediction of sales and allows us to understand what actually drives sales. For example, using statistical learning, we can also determine causal relationships and perform scenario analysis”. As an example, Etienne estimates a model that shows how a one-time 10% increase in advertising budget positively affects sales up to three months later.
Some real-life examples combining smart algorithms and analysis include using ‘spatial statistics’ to understand the best location for a new sales point, ‘financial econometrics’ to decide on when to take out a new loan based on interest rate predictions, and ‘clustering’ to create customer profiles based on loyalty cards for marketing campaigns.
However, we must be realistic about what data can tell us. Etienne concludes, “you may not have all the data you need for the best analysis; you might have made incorrect choices in the model or you may be wrong about the relationship between the variables. The good news is that smart analysis can quantify these uncertainties, such that they can be considered in your predictions and decision-making process”.
Taking the next step
If you feel our expertise on data analysis and digitalisation can help you and your organisation get ahead, please get in touch and let’s start creating an impact together!